Protease activity as a prognostic factor for wound healing in venous leg ulcers
Abstract
This is a protocol for a Cochrane Review (Prognosis). The objectives are as follows:
To determine whether protease activity is an independent prognostic factor for the healing of venous leg ulcers.
Background
Description of the condition
Venous leg ulcers (VLUs) are open skin ulcers (wounds) on the lower leg (from below the ankle up to mid‐calf), that can last weeks, months or even years, and are a consequence of problems in either the superficial or deep veins or both. Damage to the valves or vein blockages result in malfunctioning of the venous system, reducing the efficient return of blood to the heart and increasing the pressure in the leg veins (Ghauri 2010; Vlajinac 2014), which, if prolonged, may result in VLUs. The precise chain of events that links the high venous pressures with skin breakdown and a chronic wound is not fully understood (Coleridge Smith 1988; Valencia 2001). Leg ulcers are frequently associated with venous disease in combination with vascular disease, which impairs arterial blood supply, and such ulcers are said to have a 'mixed aetiology' (Marston 2011).
Accurate current estimates of leg ulcer prevalence are hard to identify because most surveys do not differentiate between causes of leg ulceration, or do so per limb but not per participant (Moffatt 2004; Srinivasaiah 2007; Vowden 2009). A recent estimate suggests that venous ulceration has a point prevalence of 0.29 cases per 1000 in the UK, whilst mixed arterial/venous leg ulceration has a point prevalence of 0.11 per 1000 (Hall 2014). A systematic review also reported the point prevalence of leg ulcers in more recent non‐UK studies: 0.39 per 1000 in New Zealand in 2004, 1.4 per 1000 in Portugal in 2005, and 2.4 per 1000 in Sweden in 2008 (Cullum 2016).
Venous disease is a chronic condition characterised by periods of ulceration (i.e. an open wound) followed by healing and then recurrence, though published contemporary data are lacking: an early cross‐sectional study reported that half of current or recent ulcers had been open for up to nine months and that 35% of people with leg ulcers had experienced four or more episodes (Callam 1987). This picture was supported by a subsequent cross‐sectional study (Nelzén 1994).
There are many prognostic factors for healing: we reviewed cohort data from 13 studies in people with VLUs (Ashby 2014; Barwell 2000; Barwell 2001; Chaby 2013; Gohel 2005; Harrison 2011; Iglesias 2004; Lantis 2013; Margolis 1999; Margolis 2004; Milic 2009; Moffat 2010; Scotton 2014) (Appendix 1). The results suggested that, in multivariable analyses, smaller initial wound area and shorter duration of ulcer were independently associated with healing (over 24 weeks of follow‐up or more); and younger age, a smaller number of wounds, mobility (especially ankle) and lower weight (or body mass index (BMI)) may also be important factors for healing.
The first‐line treatment for VLUs is compression therapy in the form of bandages, stockings or mechanical devices (O'Meara 2012). This application of external pressure around the lower leg assists venous return and reduces the pooling of blood in the legs (venous reflux) (Fletcher 2013; O'Meara 2012). Alongside compression, dressings are almost always applied to open ulcers (O'Meara 2014). Other treatments for VLUs include venous surgery (removal of incompetent superficial veins) (SIGN 2010), and drugs such as pentoxifylline (Jull 2012).
Leg ulcers are associated with considerable cost to patients and to healthcare providers.
Two systematic reviews summarised the literature on health‐related quality of life in patients with leg ulcers (Herber 2007; Persoon 2004). Both included qualitative and quantitative evaluations, and reported that presence of leg ulceration was associated with pain, restriction of work and leisure activities, impaired mobility, sleep disturbance, reduced psychological well‐being and social isolation. Ulcers can be painful, malodorous, prone to infection, and may severely affect people's mobility and quality of life (Dumville 2009; Herber 2007). In severe cases, ulceration can lead to limb amputation, though this is more likely in patients who also have arterial insufficiency (Dumville 2009; Nelzén 2008; Valencia 2001), or even to a type of cancer known as 'Marjolin's ulcer' (Choa 2015). Recent research suggests that people with complex wounds, including those with VLUs, commonly see complete ulcer healing as the most important outcome to them (Madden 2014).
The financial cost of treating a person with an open VLU in the UK has been estimated at around GBP 1700 per year at 2012 prices. A large part of ulcer treatment cost comprises nursing time (Ashby 2014). Another evaluation estimated the average cost of treating a person with a VLU in Sweden as between EUR 1332 and EUR 2585 (based on costs for material for dressing changes) and in the UK as between EUR 814 and EUR 1994 (price year 2002), with higher costs associated with larger and more chronic wounds (Ragnarson Tennvall 2005). Data from a German study, which estimated total costs including those classified as indirect or intangible costs, estimated mean annual costs of treating leg ulcers as EUR 9060 per patient (2006 prices). This figure is higher than other estimates because it includes non‐health service costs to the patient and to society (Augustin 2012). A recent Australian cost‐effectiveness study estimated the mean cost per person per week for treating 905 people with a chronic leg or foot ulcer below the knee for 24 weeks as AUD 53.31 (which corresponds to AUD 2772 per year); costs included consultations with healthcare professionals, compression bandaging, other dressings and treatments, and community care services, such as Meals‐on‐Wheels and home help (Graves 2014).
Evidence shows that not all VLUs heal, even under trial conditions: a large study in 453 participants showed that about 30% of VLUs did not heal following first‐line treatment with compression therapy over 12 months (Ashby 2014). There is interest in additional treatments that may improve wound healing, and one dressing option is the use of protease‐modulating dressings, which are suggested to reduce the activity of a group of enzymes known as proteases. As discussed below, it is thought that prolonged and elevated activity of proteases may be a feature of non‐healing wounds, such that appropriate use of protease‐modulating dressings could promote healing. However, evidence for the effectiveness of these dressings in VLUs is largely unclear (Westby 2016). Such dressings are a new development in wound care in that they are intended to be a 'targeted' treatment aimed at wounds with high protease activity. In this context, protease activity dictates subsequent treatment, but there is limited evidence supporting the use of a test‐and‐treat approach (Norman 2016). In fact, the prognostic nature of protease levels in relation to wound healing is unclear and this will be the focus of this Cochrane Review.
Description of the prognostic factor
A prognostic factor is any measure that, among people with a given health condition, is associated with a future clinical outcome; for example, in people with a VLU, lower BMI may be associated with less time to healing. The prognostic factor for this review is a biomarker, protease activity, which is sometimes elevated in open wounds. Biomarkers have been defined by the National Institutes of Health Biomarkers Definitions Working Group (1999) to be "a characteristic that is objectively measured and evaluated as an indicator of normal biological processes, pathogenic processes, or pharmacologic responses to a therapeutic intervention". Biomarkers are medical signs, as opposed to medical symptoms (which are indications of health or illness perceived by patients themselves) (Strimbu 2010). Biomarkers may predict health outcomes, but not necessarily. However, there are still no accepted definitive biomarkers for making assessments of chronic wounds (Patel 2016; Yager 2007).
One possible biomarker type that has received some attention for wounds are the proteases, which are enzymes that break down proteins into peptides and amino acids (Zhang 2012); in general, the various wound‐related proteases break down different proteins. The principal proteases involved in the wound healing process are the matrix metalloproteinases (MMPs) and the serine proteases, which break down extracellular matrix (ECM) and connective tissue proteins such as collagen and elastin (Hahm 2011; Ladwig 2002; McCarty 2013; Nwomeh 1999; Velnar 2009). This protein breakdown is thought to be important in the early stages of the healing process because it aids removal of unwanted material and bacteria. However, in the later stages of wound healing, protein breakdown is believed to be undesirable because the proteases damage newly‐formed tissue, preventing completion of healing (McCarty 2013; Velnar 2009).
Proteases are produced by an inflammatory process, which also inhibits the synthesis of chemicals that inhibit the action of metalloproteinases (Tissue Inhibitors of MetalloProteinases ‐ TIMPs). Some studies have measured the ratio of MMPs and TIMPs as a biomarker (McCarty 2013; Muller 2008), and it may be more valuable to consider the balance of proteases and their inhibitors as a biomarker (Löffek 2011; Yager 2007).
MMPs have been divided into six subtypes on the basis of the substrates they work on and their domain structure (chemical components) (Lazaro 2016; Löffek 2011; Vihinen 2002): gelatinases (MMP‐2 and MMP‐9); collagenases (MMP‐1, MMP‐8, MMP‐13); stromelysins (MMP‐3, MMP‐10, MMP‐11) and metalloelastase (MMP‐12); matrilysins (MMP‐7, MMP‐26); membrane‐type MMPs (MMP‐14, MMP‐15, MMP‐16, MMP‐17, MMP‐24, MMP‐25); and other MMPs (MMP‐19, MMP‐20, MMP‐23, MMP‐28). The main serine proteases are mast cell tryptase and chymase, plasmin, human neutrophil elastase (HNE), cathepsin G, Urokinase‐Type Plasminogen Activators (u‐PA) and Tissue‐Type Plasminogen Activators (t‐PA) (Grøndahl‐Hansen 1988). Other protease biomarkers include modular proteins that combine proteases with other biological species: for example, the families of A Disintegrin And Metalloproteinase (ADAMs) (Duffy 2009; Edwards 2008) and A Disintegrin And Metalloproteinase with ThromboSpondin motifs (ADAMTSs) (Kelwick 2015). Chronic wounds also contain proteases associated with several types of bacteria (McCarty 2012; McCarty 2013; Percival 2012; Sibbald 2007; Suleman 2016).
Protease activity can be measured in wound fluid using various biochemical tests. Laboratory‐based scientific studies have used several different techniques, including approaches that primarily detect MMP‐2 and MMP‐9 (gelatin zymography), and methods that detect enzyme activity using either chemical (e.g. quenched fluorescence substrate hydrolysis) or biological antibody‐based methods (Enzyme‐Linked ImmunoSorbent Assays (ELISAs)) (Harding 2011, McCarty 2013; Zhang 2012). A range of methods of obtaining wound fluid have been used and these vary with the type of protease measured (Cullen 2006; Quirk 2003; Trengove 1999; Yager 2007). There is also a commercial colorimetric indicator available, which is said to determine protease activity, giving a colour change if activity is elevated above a threshold; a weighted average of the activity of various proteases is used (Gibson 2014; NICE 2016).
How the prognostic factor may be related to health outcomes
It has been suggested that, in chronic ulcers generally, non‐healing may be associated with a prolonged high activity of proteases in the wound in the later stages of the wound healing process (Harding 2011; Hart 2002; McCarty 2013; Palolahti 1993).
Proteases are active in all phases of wound healing (haemostasis, inflammation, proliferation and remodelling) and are therefore thought to have a number of roles in the normal wound healing process (Patel 2016; Trengove 1999; Velnar 2009). It is thought that there is a burst of protease activity at the start of acute wound healing, and that in normally healing wounds, the activity peaks in the first few days and then declines to very low levels by one week, as healing progresses (Harding 2011; Nwomeh 1998).
In non‐healing wounds, however, it is thought that high protease activity may arise through two main routes (involving different types of proteases): relating to both the host cells (human) and to bacteria in the wound. It is thought that the two types of protease activity may reinforce each other (have a synergistic mechanism) (McCarty 2012; McCarty 2013; Percival 2012; Sibbald 2007; Suleman 2016). In the host, complex inflammatory mechanisms may result in proteases reaching higher levels and persisting for longer than in normally healing wounds (McCarty 2013; Trengove 1999). As previously noted, this persistent breakdown of proteins (proteolytic activity) is thought to damage newly formed tissue and to degrade growth factors, leading to non‐healing wounds (Cullen 2002; Harding 2011; Wlaschek 1997; Yager 1997). Most chronic wounds are colonised with bacteria, though not necessarily infected. Bacterial proliferation and their formation into film‐like material (biofilms) in non‐healing wounds has been linked to early chronic inflammation and then elevated protease levels. Infection refers to invasion of tissue by bacteria and extends the inflammatory response and tissue damage (Percival 2012; Pugliese 2016; Sibbald 2007; Suleman 2016).
Limited evidence suggests correlations between elevated levels of MMPs and delayed healing in people with pressure ulcers (Ladwig 2002), or in foot ulcers of people with diabetes (Liu 2009), as well as in people with VLUs (Mwaura 2006; Serra 2013). This may be a property of wounds more generally, but differences between wound types have also been observed (Lazaro 2016; McCarty 2013).
For VLUs in particular, studies of protease activity in wound fluid have suggested that there are significantly higher levels of proteases in ulcer tissue compared with healthy tissue, and that these levels decrease following compression treatment in wounds that heal (Beidler 2008); other studies have reported higher levels in chronic wounds compared with acute wounds (Lazaro 2016; Trengove 1999; Wysocki 1993).
However, association between protease activity and non‐healing is not clear cut. Limited data from two industry‐sponsored studies respectively found that only 28% of 162 (Serena 2011) and 23% of 139 (Gibson 2013) non‐healing wounds of mixed aetiology had high protease activity; one of these studies reported that 22% of 101 leg ulcers had elevated protease activity (Gibson 2013).
Wound fluid is a useful source of biomarkers, and its composition is broadly assumed to reflect the current clinical condition of a wound (Löffler 2013). Wound fluid can be obtained in a largely non‐invasive way, but in order to give reproducible and accurate measurement of biomarkers, the wound fluid has to be collected and processed reliably. There are several techniques for wound fluid sampling, including the use of occlusive dressings, entrapment of fluid in dressings, swabs, other techniques and devices. The duration of fluid collection can also be important (Löffler 2013).
Importance of evidence about prognostic factors
Biomarkers of this type may potentially be implicated in, or mediate, particular pathways to non‐healing. Studying the prognosis associated with biomarkers has a two‐fold purpose: firstly, it can help us understand mechanisms related to healing, including investigating the true causes of non‐healing. Secondly, it can allow identification of wounds at increased risk of non‐healing, which could allow selective treatment of these wounds according to the specific biomarker type and level. Targetted treatment of this type (the 'test‐and‐treat' approach) is important, especially if treatments are costly or have adverse effects, so that they are not used where they will not be effective. It is likely that there is more than one pathway to non‐healing and other pathways will probably be represented by other biomarkers. The best approach may be to determine a set of biomarkers and treat according to their levels.
A commercial test for protease activity is now available (Gibson 2014), and is intended for use at the point of care, in conjunction with protease‐modulating treatments (Barrett 2011; Harding 2011; Snyder 2011; Snyder 2013). This approach has mainly found application in diabetic foot ulcers (NICE 2016). A Cochrane Review on test‐and‐treat for healing in VLUs did not identify any studies (Norman 2016).
Why it is important to do this review?
VLUs are a relatively common type of complex wound that have a negative impact on people's lives and incur high costs for health services and society. Leg ulcers are painful, sometimes malodorous, prone to infection, and may severely affect patients' mobility and quality of life; in severe cases, there is a risk of limb amputation. There are a number of treatments for VLUs, but many ulcers prove hard to heal.
Two recent Cochrane Reviews investigated protease‐modulating matrix (PMM) dressings, but did not find evidence on protease activity: Westby 2016 examined the effects of PMM dressings for healing VLUs, and found it was unclear whether PMM dressings increased the probability of healing at 12 weeks, in comparison with non‐PMM dressings (RR 1.28, 95% CI 0.95 to 1.71; 4 trials, 192 participants). The study populations typically comprised people with hard‐to‐heal wounds, but the level of protease was reported only in one study. A second review searched for evidence on 'test‐and‐treat' approaches for healing VLUs; for example, PMM treatment given selectively to wounds with elevated protease activity (Norman 2016). No studies were found. The current review is the third part of this set of Cochrane Reviews on protease activity‐related treatment.
It is important to investigate whether elevated protease activity is a prognostic factor for healing. For practical use, the biomarker should be robust to adjustment for other factors such as age. This Cochrane Review is mainly exploratory in nature and its focus is on protease activity in general; it will not address associations of specific proteases, although we will report the actual proteases measured.
A recent literature review summarised clinical evidence on MMPs in chronic wound healing (Lazaro 2016). The authors reported studies that found correlations between MMP levels and various measures of healing, but did not give full quantitative data. They identified some studies investigating MMP thresholds for healing but these were in people with diabetic foot ulcers. The review did not examine the literature on proteases other than MMPs.
Objectives
To determine whether protease activity is an independent prognostic factor for the healing of venous leg ulcers.
Methods
At the outset, we note that the methods used in this review have two underlying assumptions: firstly, that biomarkers are representative of wound processes and can be used to monitor wound healing. Secondly, that the removal of wound fluid does not interfere with the healing processes (Yager 2007). It is unclear how robust these assumptions are.
Criteria for considering studies for this review
Types of study designs
We will include reports of prospective and retrospective longitudinal studies that investigate whether the prognostic factor, protease activity, is associated with healing of VLUs. We will not include studies that examine prediction models. We will include RCTs analysed as cohort studies, provided interventions are taken into account in the analysis. We will include case‐control studies if there are no cohort studies for each type of protease. We will not include cross‐sectional studies or case reports. We will include studies with any follow‐up period.
We will consider evidence separately within the different phases of prognostic factor investigation: Phase 1 (exploratory), and Phase 2 (confirmatory) studies, which provide different levels of evidence (Hayden 2008). Exploratory studies identify associations of many potential prognostic factors and outcomes. These studies measure associations between single factors and the outcome, ('univariable' or 'univariate' associations) and provide the least conclusive information regarding the independence of a variable as a valid prognostic factor. Confirmatory studies aim to measure the independent effect of a prognostic factor on the relevant outcome while controlling for other factors. We will include all eligible studies in the review but, if possible, we will restrict the results summary and any meta‐analyses to results from multivariable analyses. Failing this, we will include the results of univariable analyses and will take account of the phase of investigation in the 'Risk of bias' assessment and GRADE rating.
Types of participants
We will include studies that include people with a VLU, who are managed in any care setting. We expect the method of diagnosis of venous ulceration to vary, so we will accept definitions as used in the included studies.
We will include studies in people with VLUs, alongside people with other types of wounds (e.g. arterial ulcers, pressure ulcers, diabetic foot ulcers), provided the results for people with venous ulcers are presented separately, or if most participants (at least 75%) had leg ulcers of venous aetiology. Where wounds are described only as leg ulcers without information as to aetiology, we will assume they are venous in origin because this is the most common type of leg ulcer.
We will include studies that involve participants at any stage in their treatment pathway, and we will record, where available, baseline data on the time since diagnosis and treatments previously given, together with ongoing treatments. We will include studies that involve participants with any infection status at baseline: we will record any available data on this.
We will exclude studies conducted solely in vitro and animal studies.
Types of prognostic factors: defining protease activity
The prognostic factor will be protease activity. Any type of protease or combination of proteases will be eligible, either from the host or from bacteria, including:
-
MMPs: gelatinases MMP‐2 and MMP‐9; collagenases MMP‐1, MMP‐8 and MMP‐13; stromelysins MMP‐3, MMP‐10 and MMP‐11; metalloelastase MMP‐12; matrilysins MMP‐7 and MMP‐26; membrane‐type MMPs MMP‐14, MMP‐15, MMP‐16, MMP‐17, MMP‐24 and MMP‐25; and other MMPs MMP‐19, MMP‐20, MMP‐23 and MMP‐28;
-
serine proteases: mast cell tryptases and chymases; human nucleophil elastase; cathepsin G; plasmin; u‐PA; tissue‐activating plasminogen thrombin (t‐PA);
-
proteases combined with other biological species: ADAM; ADAMTS.
Where possible, we will combine these three major categories in the analyses, taking into consideration cut‐off points used (see 'Data synthesis' section below).
We will also include and report separately studies that investigate as biomarkers the ratio of proteases and their respective inhibitors, e.g. MMP‐2 (protease) and TIMP‐2 (inhibitor).
The prognostic factor may be examined as a continuous or categorical variable and any cut‐off point will be permitted.
We will permit any approach to obtaining samples from wounds, and any method of measurement of protease activity. We will not include measures of proteases in the blood.
We will report all unadjusted (simple) and adjusted (multivariable) associations between the prognostic factor and healing, with details on any adjustment factors used.
Types of outcome measures
Primary outcome measures
We will include the following primary outcome measures:
-
time to healing (survival analysis);
-
the proportion of people with ulcers completely healed, at any follow‐up duration.
We will consider subgroup analyses to explore the impact of follow‐up time. We will accept study authors' definitions of what constitutes a healed wound.
We will record study‐reported associations. Binary outcomes will preferably be reported as time‐to‐event measures (survival), but failing that, we will consider dichotomous summary data at the longest time point or the time point specified in the study's methods section.
Secondary outcome measures
-
Change in size of ulcer/rate of wound closure (e.g. cm²/ day).
If there are no ulcer healing data for a particular association, we will use data on the change (and percentage change) in ulcer size, with adjustment for baseline size (contacting study authors to request adjusted means when not presented). Where change in ulcer size is reported without adjustment for baseline size, we will document the use of the outcome in the study, but we will not extract or summarise data or use it in any meta‐analysis.
For continuous outcomes, we will report either continuous summary data or dichotomous data with any cut‐off point.
Search methods for identification of studies
We will search the following databases:
-
Ovid MEDLINE (1946 to date);
-
Ovid Embase (1974 to date);
-
CINAHL (1982 to date);
-
Cochrane Central Register of Controlled Trials (CENTRAL) (most recent issue).
The draft search strategy for MEDLINE is in Appendix 2.
In addition we will search the bibliographies of all included studies and of identified relevant systematic reviews.
Data collection and analysis
Study selection
Two review authors will independently assess the titles and abstracts of retrieved records against the inclusion criteria. We will obtain all potentially relevant studies in full and two review authors will independently assess these for eligibility. We will resolve any disagreements at each stage through discussion and, where appropriate, we will consult a third review author. Where there is doubt over the eligibility of a study, we will attempt to contact the study authors in order to resolve the uncertainty. Where studies are reported in multiple publications/reports, we will obtain all publications. Whilst we will include a study only once in the review, we will extract data from all reports to ensure we obtain all available relevant data. The study selection process will be illustrated in a PRISMA diagram. All studies excluded after full‐text assessment will be listed in a ‘Characteristics of excluded studies’ table with their reasons for exclusion.
Data extraction and management
We will collect all associations reported between the prognostic factor and outcomes, with details on any adjustment factors used.
We will extract and record data from included studies using a data extraction sheet. One review author will extract data, which will then be checked by a second review author. If key data are missing from reports we will attempt to contact the study authors.
We will extract the following data for the pre‐specified prognostic factors and outcomes in this review. We will collect outcome data as described in the ‘Types of outcome measures’ section.
We will extract the following data:
-
country and setting in which study was conducted;
-
study design;
-
eligibility criteria;
-
participant details;
-
ulcer details, including duration of ulcer, ulcer size, number of ulcers and ulcer history, and ulcer severity (with the system used to classify this) as reported by the study authors;
-
treatment details, including compression and debridement (including type and frequency);
-
method of obtaining wound fluid for prognostic factor measurement, including duration of collection;
-
method of measurement of protease activity (e.g. Gelatin zymography);
-
details of each prognostic factor: type of protease/combination of factors, measurement of prognostic factor, type of data (e.g. continuous/any cut‐off points and if so, whether they were pre‐defined and what the justification was for that cut‐off point);
-
details of each outcome: measurement, type of data (e.g. continuous/any cut‐off points);
-
duration of follow‐up;
-
type of analysis: e.g. explanatory/confirmatory (including the presence of a pre‐defined protocol and study registration); logistic regression/Cox regression;
-
any adjustment factors considered in the analysis;
-
association statistics for each prognostic factor for primary and secondary outcomes (e.g. odds ratios (ORs), hazard ratios (HRs) and their confidence intervals (CIs)/variances/standard errors);
-
loss to follow‐up, and reasons.
Assessment of risk of bias in included studies
Two review authors will independently appraise the included studies using a standardised approach. In the case of discrepancies, the review authors will attempt to reach consensus; if necessary a third review author will resolve any disagreements. The review authors will not be blinded to study authors, institution or journal of publication due to feasibility.
We will assess risk of bias using an approach based on the Quality In Prognosis Studies (QUIPS) tool, which is appropriate for prognostic factor review questions (Hayden 2013; Hayden 2014). Our approach also draws on the ROBINS I tool (ROBINS‐I 2017) which assesses the risk of bias for non‐randomised intervention studies (Sterne 2016). We have provided further details in Appendix 3. This approach assesses risk of bias per study for each prognostic factor‐outcome combination, by considering six domains: study participation (selection bias), study attrition, prognostic factor measurement, outcome measurement, adjustment, and statistical analysis and reporting. Each domain is rated as having high, moderate or low risk of bias. We will define an all‐domain risk of bias per study for each prognostic factor‐outcome combination, taking into account all of the above domains, but especially focusing on the key domains of selection bias, study attrition, adjustment, and statistical analysis and reporting. As a guide, we will assign risk of bias as follows: low risk of bias if all (or all key) domains have low risk of bias; moderate risk of bias if there is high risk of bias for one key domain or moderate risk of bias for at least two key domains (with the rest as low risk of bias); high risk of bias if there is high risk of bias for at least two key domains. We will perform sensitivity analysis considering only studies at low all‐domain risk of bias (Hayden 2013).
Measures of association
We will extract all unadjusted and adjusted measures of association from included studies and convert effect sizes, as necessary, in order to avoid possible selection bias, thus allowing us to use data from as many studies as possible. HRs are the preferred measure of the relationship between individual prognostic factors and healing outcomes, and we will separately extract and analyse HRs for studies providing this measure of association. However, we anticipate that results will also be reported as ORs and RRs; we will use ORs as the common measure of the association, and RRs and HRs to estimate ORs at a particular time point (Symons 2002).
For consistency, we will re‐calculate associations to be in the same direction, as necessary, with associations above 1 indicating better prognosis for positive binary outcomes (e.g. a healed wound). We will appropriately transform the individual study associations (e.g. ORs) and their 95% confidence limits to their natural logarithms to normalise their distributions and then will calculate the standard error. We will contact study authors regarding missing or unusable data, as necessary.
Where the outcomes are reported as continuous measures (e.g. change in ulcer size), we will analyse the regression coefficients with their standard errors.
If we cannot obtain OR values from the published papers or from the study authors, we will report correlation coefficients or any other measure of association.
Unit of analysis issues
The prognostic factor (protease activity) and outcome (complete wound healing) are both considered at the ulcer level. A possible unit of analysis issue could arise when there is more than one ulcer per person and multivariable analysis has been conducted with adjustment factors measured at the individual level (e.g. patient age). This represents clustered data and analyses should be conducted using hierarchical methods. Where studies include clustered data of this type we will report this, noting whether data are analysed correctly. We will record this as part of the 'Risk of bias' assessment. We will consider including such studies in any meta‐analysis, taking into account the associated risk of bias.
Dealing with missing data
We will include studies that investigate the relationship between protease activity and healing regardless of whether or not there are missing data and even if limited evidence is provided about the size of the effect (for example, if the factor is mentioned only as being ’non‐significant’ in the analyses). If necessary, we will contact study authors for clarification and to attempt to retrieve any missing information.
Assessment of heterogeneity
We will consider the clinical heterogeneity of included studies based on the population, measure of the prognostic factor, cut‐off points used, outcome measurement and methodological heterogeneity due to study design/potential biases. We will synthesise associations as appropriate within clinically‐relevant subgroups, grouping studies regardless of type of protease, duration of ulcer and presence of infection. We will examine the latter two features in subgroup analyses where there is heterogeneity.
Reporting bias
We will examine publication bias for each meta‐analysis, provided there are 10 or more studies, by visually examining asymmetry on funnel plots and testing for asymmetry at the 10% level, using Egger’s test for HRs, and Peters’ test for ORs (Sterne 2011).
Data synthesis
Synthesising data in prognostic factor studies requires us to recognise the many different ways of reporting and analysing results, and, where possible, to request alternative data or to transform results to a common format (see 'Measures of association' section). We will consider the impact of data transformations using sensitivity analyses.
We expect that most studies will measure and present data about prognostic factors for the healing of VLUs, such that the outcome variable is analysed as dichotomous data, but the prognostic factor may be reported as continuous data (e.g. protease activity) or values may be dichotomised with different cut‐off points or there may be more than two categorical levels to the prognostic factor.
After carrying out any appropriate transformations, where possible we will group together studies with similar prognostic factor cut‐off points/similar analytical approaches, and will represent results for different analytical approaches as subgroups on a forest plot for that prognostic factor‐outcome combination, even if we do not conduct a meta‐analysis. Where possible and appropriate, we will conduct meta‐analyses. We will include in the forest plots details of any adjustment factors considered in the study analyses.
We will conduct meta‐analysis if valid data are available assessing associations between individual prognostic factors and an outcome of interest for sufficiently homogeneous subgroups of studies. We will define 'sufficiently homogeneous' subgroups according to population, measures of the prognostic factor and outcome measurement.
For prognostic factors that are analysed as continuous measures, we will combine the data for all proteases, regardless of the source or type of protease; for prognostic factors analysed as dichotomous measures, we will take cut‐off points into consideration as described above. We will analyse separately ratios of proteases and their inhibitors.
We will conduct separate meta‐analyses of HRs and ORs (at similar follow‐up points), and will also transform the measures, where possible, so that one analysis (OR) can be conducted. We will conduct meta‐regression analysis if there are more than 10 studies providing sufficient data.
We will conduct meta‐analyses using STATA (StataCorp version 14) with a random‐effects restricted maximum likelihood (REML) meta‐analysis model, which accounts for any between‐study heterogeneity in the prognostic effect (Cornell 2014). Such heterogeneity is common in prognostic factor studies. Unless the heterogeneity (as assessed above) is too extensive for appropriate pooling, we will summarise the meta‐analysis by the pooled estimate (the average prognostic factor effect), its Hartung‐Knapp 95% CI, the estimate of Tau² (between‐study variance), and a 95% prediction interval for the prognostic effect in a single population (Riley 2011).
'Summary of findings' table and GRADE assessment
We will use an approach modified from the GRADE framework (Guyatt 2011a) to assess the overall quality (certainty) of evidence for each prognostic factor‐outcome combination (Hayden 2014;Iorio 2015). We will rate the overall strength of evidence as either ‘high’, ‘moderate’, ‘low’ or ‘very low’ considering the phase of prognostic study (confirmatory/explanatory or exploratory), the within‐study risk of bias, the directness of evidence, heterogeneity, precision of effect estimates and risk of publication bias (Iorio 2015;Schünemann 2011b). We will also consider two 'upgrading' factors ‐ large effect and dose effect ‐ although we note that high risk of bias may artificially lead to large effects (see Appendix 4 for further details).
We will present the main results of the review in 'Summary of findings' tables. These tables present key information concerning the certainty of the evidence, the magnitude of the associations examined, and the sum of the available data (Schünemann 2011a). 'Summary of findings' tables also include an overall grading of the evidence. This defines the certainty of a body of evidence as the extent to which one can be confident that an estimate of effect or association is close to the true quantity of specific interest.
We will present evidence for the various prognostic factors in rows in 'Summary of findings' tables.
If it is not appropriate to combine results using meta‐analysis (too much clinical heterogeneity), we will present the results qualitatively, considering the strength and consistency of results using the following schema:
-
strong evidence of effect: consistent findings (defined as > 75% of studies showing the same direction of effect) in multiple low risk of bias studies;
-
moderate evidence of effect: consistent findings in multiple high risk of bias and/or one study with low risk of bias;
-
limited evidence of effect: one study available;
-
conflicting evidence of effect: inconsistent findings across studies;
-
no effect: no association between patient expectations and the outcome of interest.
We will calculate absolute risk differences for the effect of the prognostic factor using estimates of baseline risk from the literature where possible.
Subgroup and sensitivity analyses
We will use sensitivity analyses to explore the impact of study all‐domain risk of bias, firstly restricting the analysis to studies rated as having low risk of bias, and if this is not feasible, restricting to low or moderate risk of bias.
If there is heterogeneity, we will investigate it using the following pre‐specified subgroup analyses, provided there are at least two studies per subgroup:
-
baseline duration of ulcer (up to 24 weeks; ≥ 24 weeks); duration may be a proxy for a non‐healing wound, in which protease activity may be associated differently with healing;
-
presence or absence of infection.
We will not conduct subgroup analyses by type of protease because this would introduce too high a level of complexity for this review, but will record the type of protease measured.
We will consider subgroup or sensitivity analyses to explore the impact of types of measurement approaches for assessing prognostic factors.
This Methods section is based on the exemplar Cochrane prognosis review protocol for prognostic factors (Hayden 2014).

Prognostic factors for healing VLUs: person characteristics
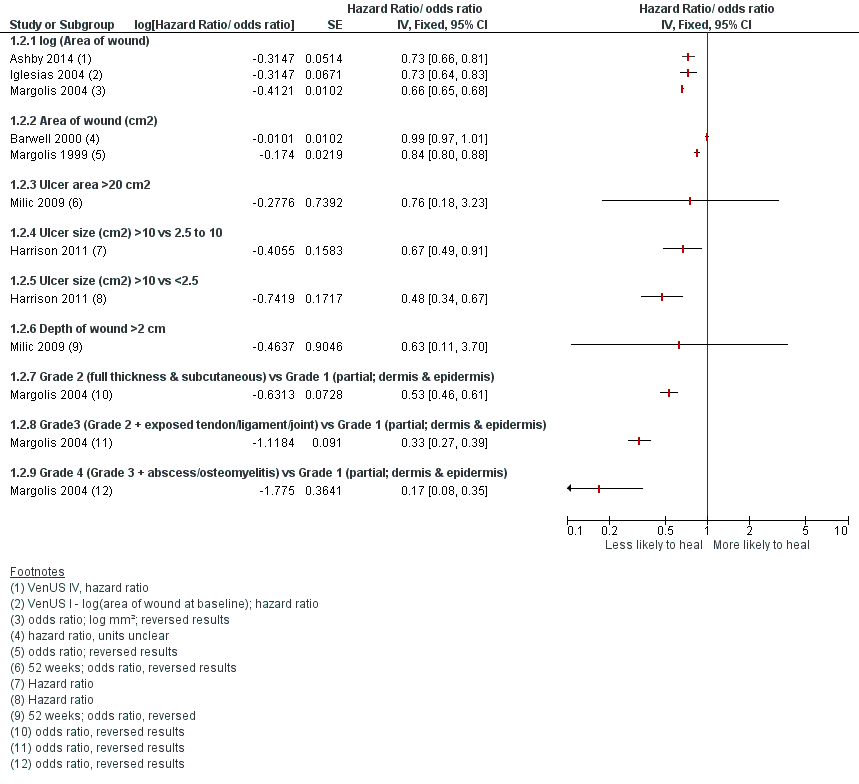
Prognostic factors for VLU healing: wound characteristics 1

Prognostic factors for VLU healing: wound characteristics 2
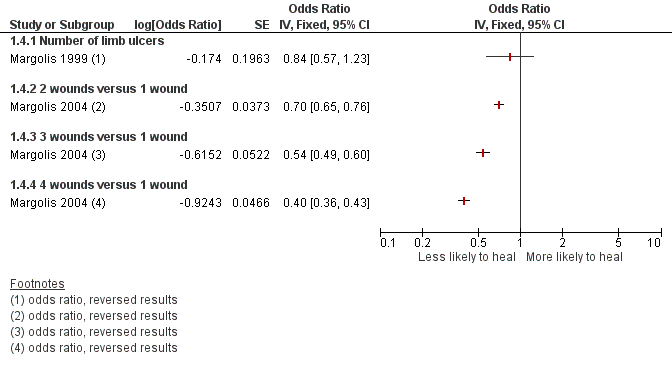
Prognostic factors for VLU healing: number of ulcers
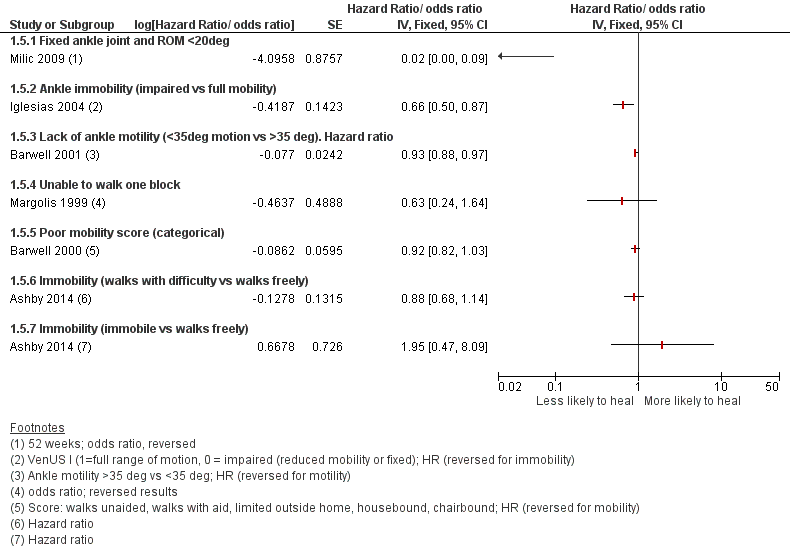
Prognostic factors for VLU healing: mobility and ankle flexion

Prognostic factors for VLU healing: bacteria and infection